Modernizing your data stack is just as critical for startups as it is for enterprises. Data analysis drives the foundational business decisions your startup depends on, minimizing the risk of failure and helping you achieve sustainable business growth. From small-scale customer appreciation campaigns to the largest
online marketing platforms, everything is built on robust analysis of data.
For large businesses and enterprises, modernizing your data stack is essential for keeping up with customers who expect ever-more personalized and efficient customer experiences.
This article explores how and why you should modernize your data stack to reap business intelligence (BI) insights. But first, let’s explore the different phases of data stacks in the frame of your company’s maturity, budget, current resources, and goals.
4 Phases of Data Stacks
For clarity, data stack maturity can be condensed into four key stages:
- The starter stack
- The growth stack
- The machine learning stack
- The real-time stack
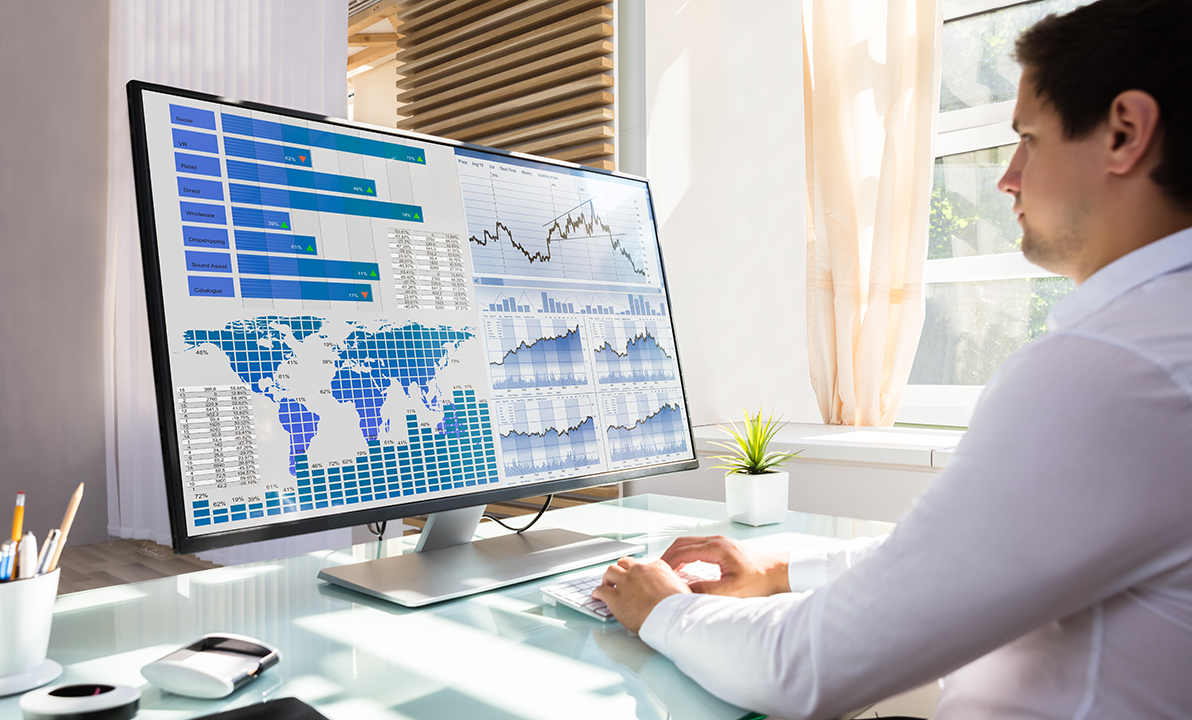
Starter stack
The starter stack is a simple data architecture used by startups or small businesses at the beginning of their data maturity journey. It focuses on collecting data from your main data sources (such as websites, social media, or the AI app development process) and transferring it to downstream landing places, such as a data analytics platform.
The starter stack facilitates better reporting. Marketers, for example, can send website data to Google Analytics to visualize the result of their SaaS SEO strategies or mobile optimization implementations. Content managers may collate open and click-through rates as part of their email marketing strategy to improve customer engagement. A manager can collect internal KPIs to report on employee performance.
Starter stacks enable startups with limited budgets and low volumes of uncomplicated data to unify multiple systems and reduce the painful complexity of point-to-point integration. The starter stack is the most critical part of your data journey as it lays the foundations for the future of your data maturity.
However, as your data volumes grow, starter stacks can present a variety of challenges. These include impaired website and app performance, increased integration complexity, and the inability to support bi-directional data flows as tools and technologies expand.
Growth stack
The growth stack phase resolves some of the most pressing challenges of the starter stack, challenges that are inevitable once your data swells in volume.
The growth stack’s main goal is to centralize data management within a data warehouse. A data warehouse is a digital storage repository for structured and semi-structured data (predominantly historical data). It can pull data from various disparate systems and create bi-directional data flows, making them a critical stepping stone for removing data silos and creating a single source of truth.
It’s similar to the “single pane of glass” concept in that data from lots of separate devices is consolidated into one solution.
A warehouse-centric structure begins to facilitate data mining and permits companies to build customer journeys and profiles. With increased data accessibility and uniformity, users can run queries and analyses more successfully.
At the growth stack stage, you’ll need a team of data analysts to perform data cleansing, interpretation, and visualization.
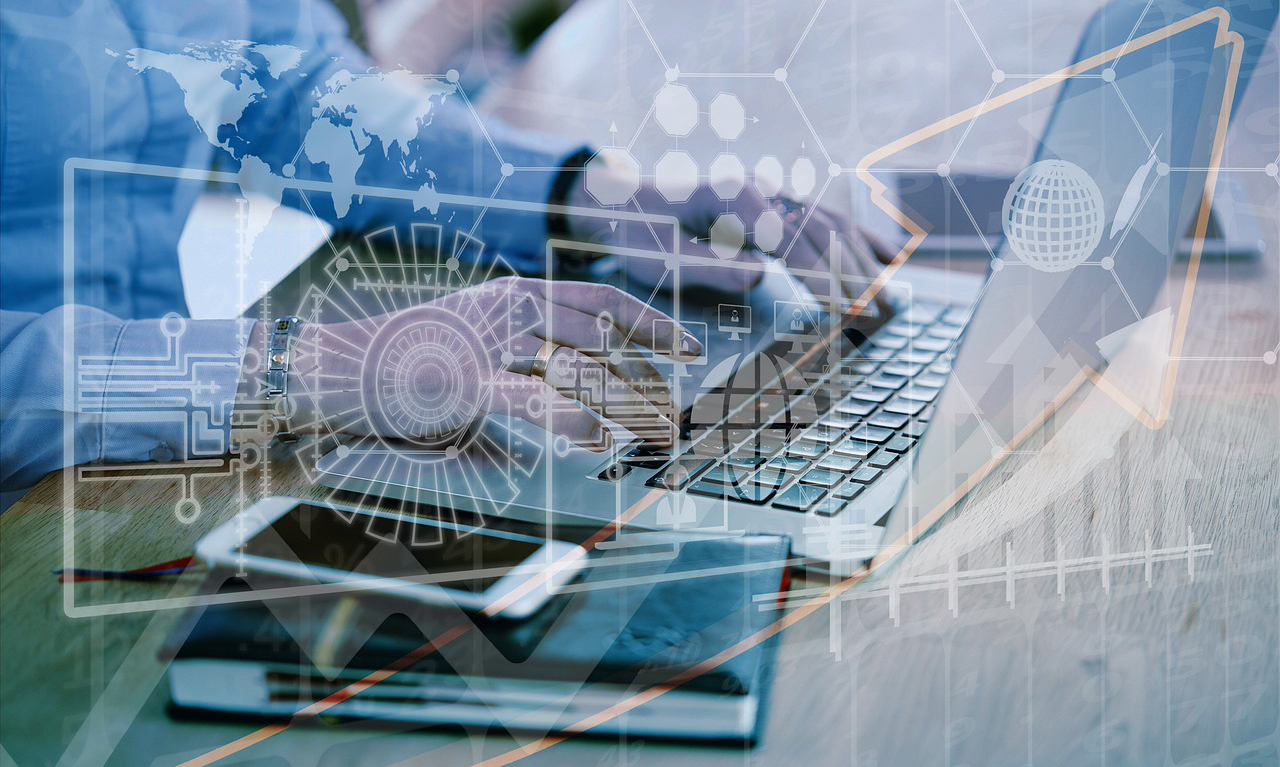
Machine learning stack
Once a data warehouse has been centralized into your data architecture, it’s time to move on to the next phase of data maturity with a data lake.
Data lakes have the ability to store vast pools of structured, semi-structured, and unstructured data. Raw, untransformed data is highly malleable, optimal for machine learning, and facilitates different types of data analytics, most notably predictive analytics.
Predictive analysis utilizes data, modeling, and machine-learning techniques to forecast potential outcomes pertaining (but not limited) to user behavior.
Let’s say you wanted to prevent customer churn as part of your post-purchase customer retention strategy. Predictive analytics would use historical customer data to pinpoint where customer churn commonly occurs in the customer lifecycle. From there, you can identify customers at risk of churn and target them at the optimal time.
Machine learning and data engineering resources are heavily invested in and optimized at this stage. Data mining and analytics are being used to inform future business outcomes and drive business transformations, albeit with varying levels of sophistication.
Real-time stack
As you conduct competitive product analysis, you’ll likely discover that most businesses comfortably mature (or aim to mature) to the ML stack phase. However, global enterprises with millions of customers might require outputs to be sent from the warehouse directly back to the application.
Why? To enable them to deliver personalized customer experiences in real-time.
With advanced machine learning techniques and an online in-memory data store, real-time stacks can modify and customize a user’s website or app experience based on offline and online predictions.
The Benefits of Modernizing Your Data Stack
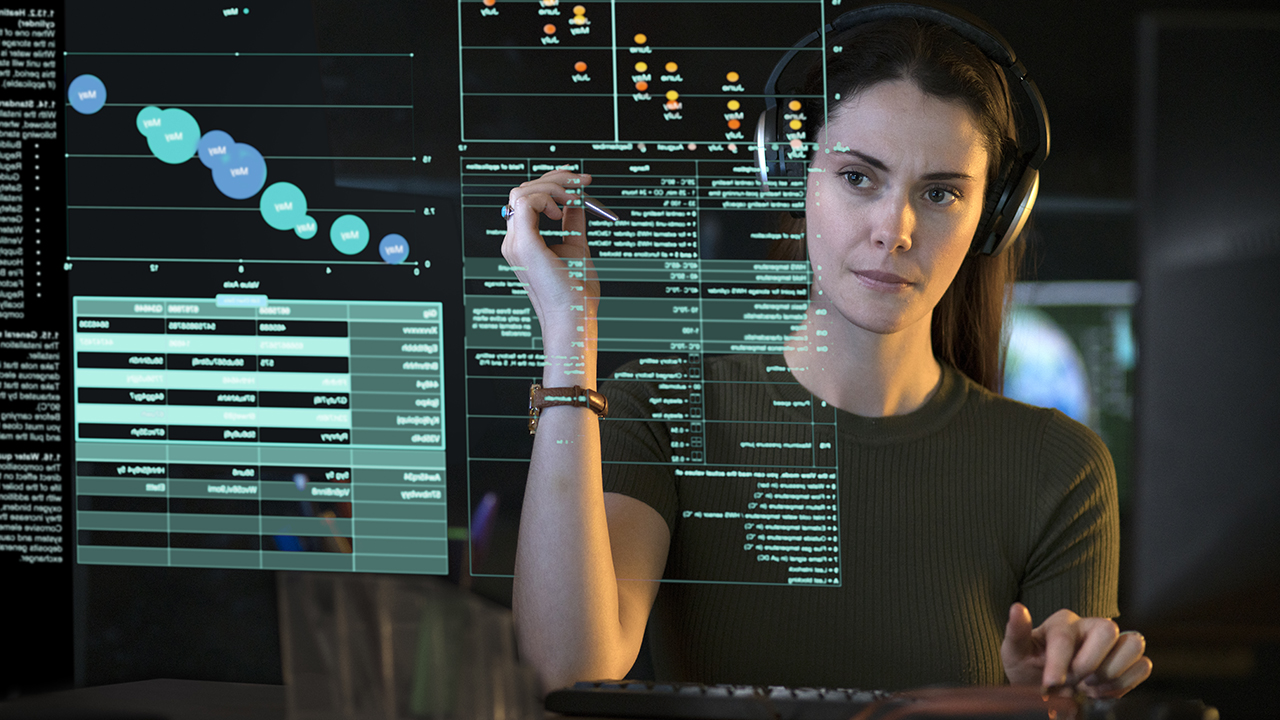
As we’ve mentioned above, one of the most valuable benefits of data stack modernization is that it improves the productivity and accuracy of data analytics. According to a recent study by MicroStrategy, usage of analytics has resulted in an abundance of benefits, including increased productivity, faster decision-making, and better financial performance. By streamlining the customer journey, it can speed up the process of getting paid, made easy.
Standardizes the team’s understanding of the data
Modernized data stacks are always centralized and unified. They use data warehouses, data lakes, and other tools and integrations to remove data silos and create a single source of truth.
By ensuring that disparate departments and remote working teams have access to consistent data, you empower teams to collaborate productively and make unified business decisions.
Can be done without many resources
Data stack modernization doesn’t require you to invest in lots of expensive, complex resources. In fact, data warehouses and data lakes are the two main resources that facilitate modernization, along with your BI tools and the talent you align with them.
Reduces costs
Cloud data warehouses and data lakes are low-cost storage solutions whose scalability and flexibility can significantly reduce costs. Beyond this, modernization powers up your data stack with new capabilities. With the freedom to improve internal workflows and data quality, you can accelerate the profitability of your analytics.
Opens the way for faster project execution
The faster you complete projects, the more valuable insights and innovations you can act on. Modernized data stacks increase data visibility and drive organizational efficacy, both of which work in tandem to lead your teams toward better project management and fast project execution.
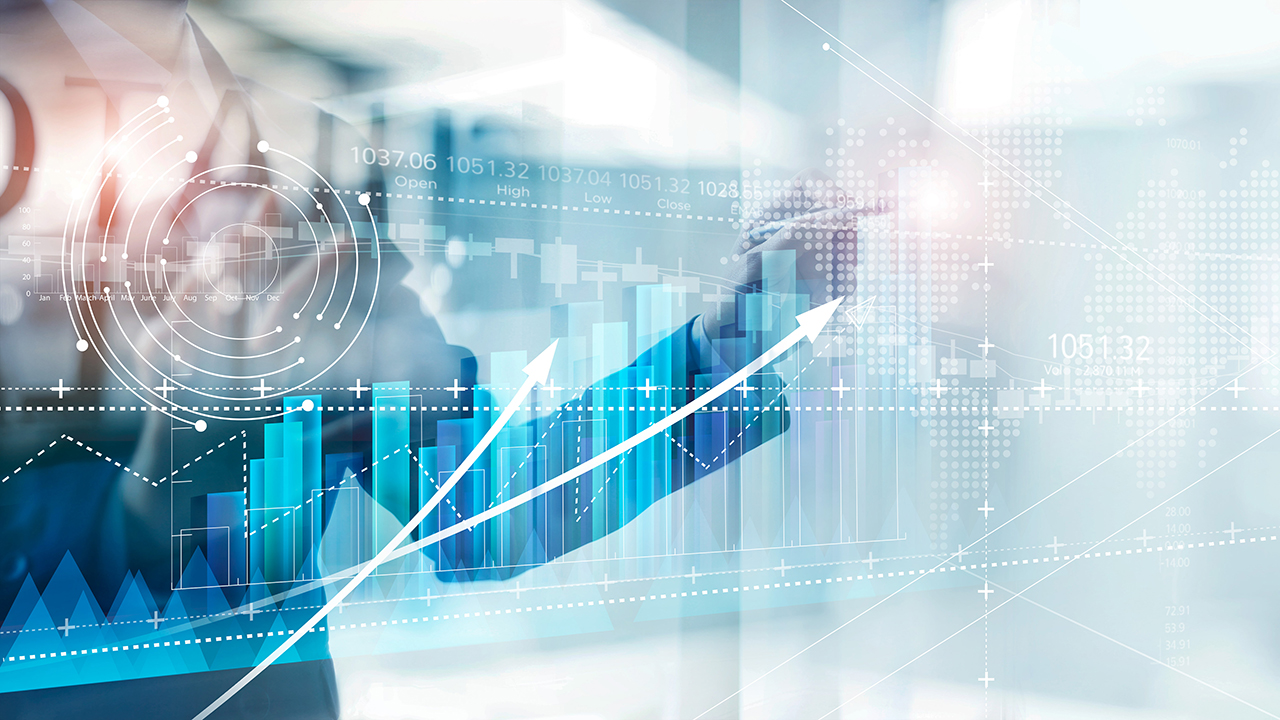
5 Steps in Setting Up a Modern Data Stack
The competitive advantages gained from a data stack modernization strategy are enormous. But, of course, modernization doesn’t happen overnight. Here are five essential steps you should take when setting up your modern data stack.
1. Determine data sources
Where is your data coming from, and which sources/data types are relevant to your business goals?
Identifying the right data sources is a key step in building a foundation of data visibility and reliability. If you’re using Java web scraping tools, make sure you use the best available. Knowing where all of your data comes from prevents invisible data from damaging the accuracy of your insights.
Also, if there’s data you need but don’t yet have access to, make sure you invest in the appropriate ecommerce tools and lines of business before moving on to the next stage.
2. Select a data warehouse fit for your business
Data warehouses differ in their specs, features, benefits, and priorities. Do your research before committing to a data warehouse provider. The most popular solution may not be the best fit for your unique business needs.
Some things to consider when you’re choosing a data warehouse include:
- Data volume and types
- Ease of scalability
- Security
- Time-to-value
- Initial setup costs and pricing structure
- Ongoing maintenance costs and resource access
- Performance
- Support
It’s also worth considering whether a data lake (or even a next-generation technology like a data lakehouse) is a better fit for your business.
Data lakes are essential for companies that collect large volumes of unstructured data. They also enable stricter data governance and quality, enabling businesses to manage and reliably communicate everything from these critical audit matters examples to GDPR and CCPA regulations.
3. Choose a data ingestion tool to use
Your ETL (extract, transform, load) process needs to be efficient if you want to quickly derive value from extracted data. Data ingestion tools handle this process, collecting data from your data sources and importing it to your storage solution.
4. Data modeling
Data modeling is perhaps one of the most complex tasks within a modern data strategy. It involves analyzing data to identify and define relationships, using these insights to create simplified data models that act as visual representations of your data flows.
To successfully perform data modeling, it’s likely that you’ll require the assistance of a data or analytics engineer with dbt knowledge.
5. Data activation or data operationalization
Data activation (a.k.a data optimization) is how data is made actionable for end-users without needing resource-intensive processes.
You can think of data activation as a “reverse ETL” process. This is when data is extracted and transformed within a data warehouse and loaded onto third-party business applications (accelerate agency SaaS tools, CRMs, etc).
Data activation modernizes your data stack by delivering self-service to end users. Instead of relying on IT and science teams for data insights, non-technical teams can easily access and utilize actionable data to independently drive data-driven initiatives.
At Digi, the Digi Remote Manager® software-as-a-service solution manages the entire IoT stack.
Final Thoughts
Data stack modernization centralizes and consolidates data for increased visibility and accessibility. But it isn’t enough to make a habit of hoarding data—it's what you do with it that counts.
By investing in your data stack, you can spend less time engineering data pipelines and more time conducting data analytics. The deeper you dive into data analysis, the more opportunity you have to uncover powerful business intelligence insights. It also gives you more time to focus on other growth strategies, like utilizing AI in content marketing.
So, it doesn’t matter whether you’re in the starter phase or the machine learning phase. As long as you’re taking steps to continuously modernize your data stack, you’re on the right path to success.
Next Steps
About the Author
Nick Brown is the founder & CEO of Accelerate Agency, an SEO agency based in Bristol.
He has over 12 years experience in digital marketing and works with large companies advising them on SEO, CRO, and content marketing. He has written for sites like HubSpot and BambooHR.